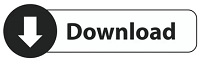
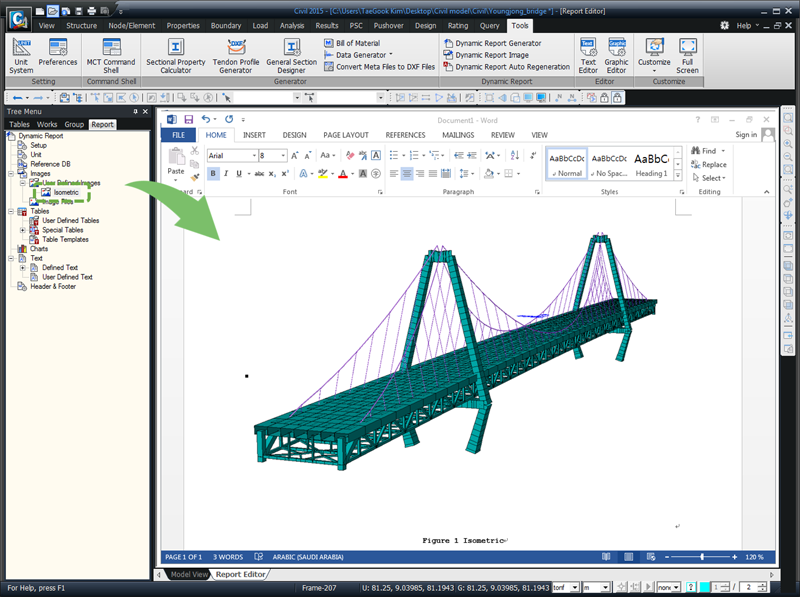
Note that the way we connect layers is computational efficient.

Many evidences (like this and this) validate that the SD encoder is an excellent backbone. In this way, the ControlNet can reuse the SD encoder as a deep, strong, robust, and powerful backbone to learn diverse controls. Stable Diffusion + ControlNetīy repeating the above simple structure 14 times, we can control stable diffusion in this way: Why "zero convolution" works?Ī: This is not true. Q: But wait, if the weight of a conv layer is zero, the gradient will also be zero, and the network will not learn anything. This is also friendly to merge/replacement/offsetting of models/weights/blocks/layers.

This allows training on small-scale or even personal devices. The "zero convolution" is 1×1 convolution with both weight and bias initialized as zeros.īefore training, all zero convolutions output zeros, and ControlNet will not cause any distortion. Thanks to this, training with small dataset of image pairs will not destroy the production-ready diffusion models. The "trainable" one learns your condition. It copys the weights of neural network blocks into a "locked" copy and a "trainable" copy. Official implementation of Adding Conditional Control to Text-to-Image Diffusion Models.ĬontrolNet is a neural network structure to control diffusion models by adding extra conditions. Those new models will be merged to this repo after we make sure that everything is good. News: A nightly version of ControlNet 1.1 is released!ĬontrolNet 1.1 is released.
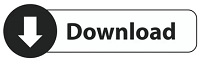